Data-driven attribution has reigned prominent among marketing buzzwords for years. While mentioned across many conference rooms during future planning meetings, many organizations have yet to truly embrace the technology given its inherent complexity, historically high costs, and difficulty in winning long-term adoption as a source of truth for meaningful decision-making. However, many of the hurdles to accessing this software have been leveled in recent years as the technology has never been more affordable, usable, and teachable.
What is data-driven attribution?
Marketing attribution has existed in some form since advertising was invented. It aims to answer the question: Which of my ads are doing the most to win-over prospective customers?
Attribution has evolved mightily since the late 19th century when John Wanamaker famously remarked: “Half the money I spend on advertising is wasted; the trouble is I don’t know which half.”
Today, the ability to measure brand interactions and ad exposures across the digital and traditional ecosystem has never been easier, yet questions about true attribution still persist. While it’s easy to track which ads were seen by newly acquired customers, the question of which ad(s) were most effective often remains unknown. Data-driven attribution aims to answer this question.
Many marketers still rely heavily on subjective attribution models; the most common of which is the last-touch model. With this methodology, if your prospect was exposed to three different ads prior to becoming your customer, whichever ad they saw last gets credit for the acquisition. The two preceding ad exposures go largely uncredited.
Some marketers have taken the next logical step forward to utilize more dynamic subjective attribution models — sometimes giving full credit to the ad credited with the first exposure, sometimes dividing credit equally between each ad in the sequence, and sometimes even weighting credit based on timing or position in the ad exposure sequence. These more evolved methodologies still fall short of the full picture, however, as they’re all inherently subjective. At the end of the day, a person’s subjective opinion of which ads deserve the most credit (or which model to choose) still obscures the most objective answer of which ads are the most effective (agnostic of their position in the sequence).
The most evolved models we have today involve data-driven attribution. This methodology relies on mathematical models to objectively distribute conversion credit not based on position but on which ads truly did the most to motivate your prospect to become your customer.
Different data-driven attribution providers rely on different mathematical models to compute their results, but most utilize some form of advanced regression or pathing analysis.
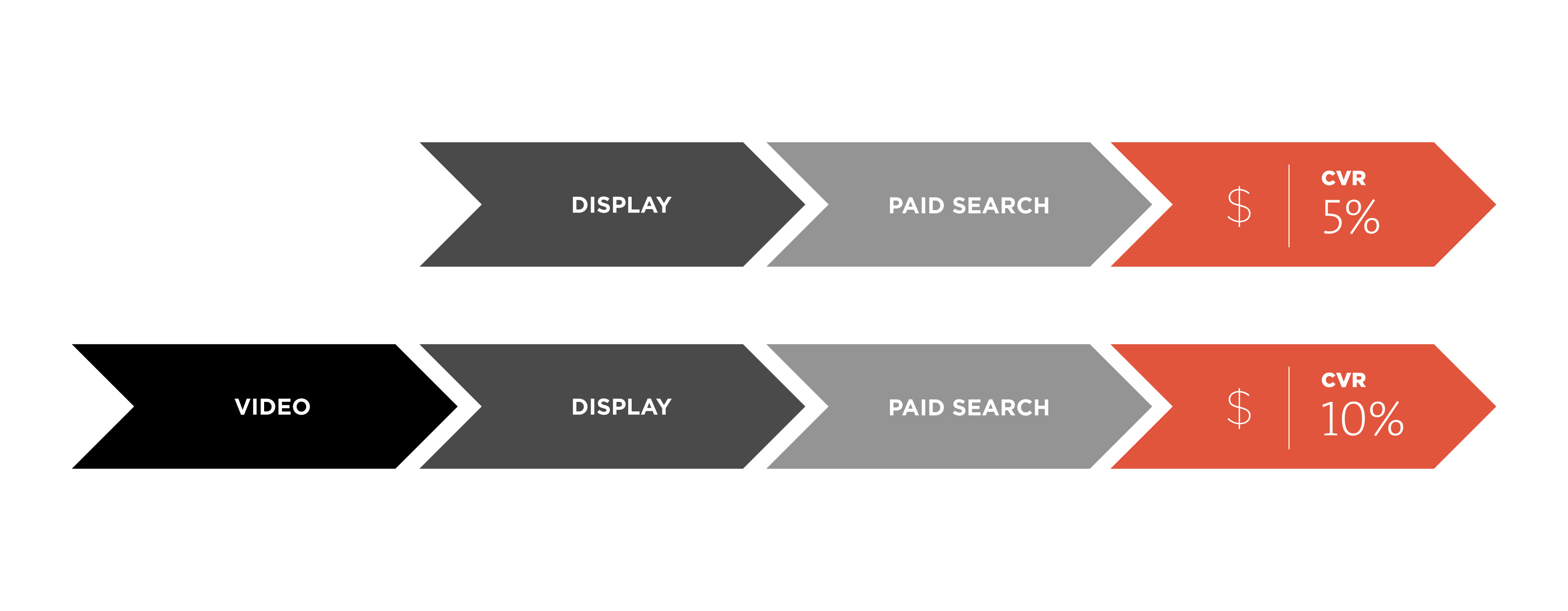
Illustrated above, the top row depicts a path where prospects are exposed to two ads (first a display ad, then a paid search ad) prior to converting. In this example, all the prospects who shared this sequence in common ultimately converted to customers at a rate of 5%.
The second row shows a slightly different sequence, where these prospects interacted with three ads, initiated by a video ad exposure, and then followed by a display ad and a paid search ad. The data-driven attribution model recognizes the difference in these common paths (specifically the addition of a video ad exposure) as well as the difference in conversion rates. Since prospects following the second path, which includes a video ad exposure, are twice as likely to convert as prospects following the first path, the model reports that the video ad must be the reason that the likelihood of conversion is doubled. As a result, the model concludes the video ad deserves the greatest amount of credit for these types of conversions. The data-driven attribution model objectively quantifies and answers the question of which ad did the most to truly motivate a prospect to become a customer.
Using these models removes subjectivity from the equation, and ultimately prescribes an ROI for each ad based entirely on its inherent effectiveness in driving a prospect to convert. These models value incrementality above all else; they ensure the ads that do the most work get the most credit, algorithmically.
Where can I access a data-driven attribution model?
Years ago it could cost upwards of $1MM per year to utilize a data-driven attribution model. The example of pathing analysis above considers only two different paths when, in reality, all your customers likely took thousands of different potential paths prior to converting — which makes the calculations incredibly process-intensive, taking expensive computers an extremely long time to compute.
Today, however, industry titans have driven down the cost of this technology to make some versions of data-driven attribution tools even free to use. Data-driven attribution tools now come free with Google Analytics 4 and a variety of other platforms.
Free data-driven attribution tools do come with limitations — they often only consider digital data and often employ data sampling to minimize the required computing resources. Data-driven attribution tools that consider offline ad exposure (TV, radio, billboards, etc.) and those that avoid sampling to improve accuracy can still cost a pretty penny, but their fees are still much more accessible for mid-to-enterprise-level companies than they had been in years prior. Their value in supporting data-driven decision-making as it relates to the ad and campaign-level optimizations is unrivaled.
Will data-driven attribution answer all my attribution questions?
The short answer is no. Employing a data-driven attribution model in your organization has never been more paramount. And utilizing these tools on an ongoing basis with broad stakeholder buy-in to inform meaningful decisions should be a primary goal of any modern marketer. While the outputs of your data-driven model should be highly valuable, they do come with some limitations.
Data-driven attribution models require a significant amount of conversion data in order to be effective. You’ll likely need to amass thousands of conversion records in order to effectively feed the model to the point where it can produce meaningful and actionable results.
As a result, the output of your data-driven model likely isn’t something you can rely on for day-to-day, or even week-to-week optimizations or decisions. It’s often more meaningful to evaluate these results on a monthly or quarterly basis (and potentially even less frequently depending on the volume of data and the granularity you expect of the results).
This means there’s still value in subjective attribution models as well, especially when it comes to daily or weekly decision-making, as these models, though imperfect, have the benefit of providing results given much lower volume thresholds. Therefore the ideal use of attribution tools requires some form of hybrid solution, where subjective models are used for more frequent tactical decisions, while data-driven objective models are used for less frequent, more strategic decisions.
Data-driven attribution won’t solve all your problems, and it certainly won’t make every decision for you, but it should be the backbone of your marketing optimization strategy. Fortunately, it’s never been more approachable.